I would like you to imagine a world where some people are sick and tired of elevators and escalators. They are, according to a small but rapidly-growing group of evangelists, simply too inefficient.
Hundreds of thousands of work-hours are being wasted as people idle on these contraptions, and there just has to be a better way.
Their proposed alternative is jumping from windows and roofs of office buildings with parachutes, and it is catching on very fast.
New companies are being spooled up, selling low-altitude-jump parachutes to office workers all throughout central business districts, and a small but significant subset of people are buying in.
After all, it's worth a shot, and the things are so damn cheap. Far cheaper than the maintenance costs of an elevator, and far cheaper than parachutes usually are (don't worry about how sustainable this low pricing is).
There are risks, of course (not that anyone selling these parachutes would easily admit so). They're framed as not very significant: even a well-maintained elevator is likely to experience maintenance issues twice a year (an almost 1% chance of issues per day), whereas BASE jumping has an incredibly low risk rate of around 0.02%: that's around five times less risky!
Occasionally, someone splatters on the sidewalk. Advocates are quick to point out that they simply didn't perform their safety checks properly before jumping (never mind that doing so is an acquired skill, training for which is not included in the purchase of a parachute).
Besides, hundreds of millions of dollars are being poured into research on better and better parachutes.
Some people have concerns about insufficient training, the fundamental limits of human reaction times, and a lack of safe landing zones in city streets.
Oh, and there's the non-trivial problem that physics puts fairly hard limits on how fast you can slow yourself down with a parachute, so some jumps are basically impossible to do safely.
But it's okay: some people insist research into stronger and bigger parachutes will solve all these problems. Especially the 'physics says we can't safely slow down faster' thing. That's the most solvable part, apparently. Or it will be, eventually.
A non-trivial chunk of the economy becomes heavily invested in parachute sales and production. Reputable textile scientists are dabbling in the field and publishing literature on BASE jumping.
Scientists are hopping in from other fields to also publish papers, because why not? They understand physics, so clearly they're qualified to publish papers recommending BASE jumping be used in offices all around the world.
Otherwise unrelated companies in textiles, vertical movement machinery, and even construction equipment are also diversifying into the BASE space; it is, after all, the future. Just try it, they'd say. It's the future! You'll love it. It makes life so much easier.
Future.I would like you to imagine how absolutely lost you'd feel, transported into this world, desperately trying to convince any of these people that BASE jumping is not a feasible alternative to fucking elevators.
For one, how the hell are you supposed to go UP? How has nobody taken that part seriously? Why are they taking the elevators out of your 50-floor office building and replacing them with untested 'indoor BASE jumping chutes'?
What is going on, and who is going to bear the cost of elevator re-installation when this stupid fad finally dies out?
The sheer number of ways in which this idea is astoundingly bad is so large it's hard to know where to start.
Fully immersed in that hypothetical scenario?
Great. Now you know how I feel any time people say generative machine learning software should be used in literally any real-world application where accuracy and safety are important. Like healthcare.
This is Not Sensible
There is a considerable amount of economic and logistical pressure to reduce the amount of human labour required in healthcare. After all, if we are aiming to save and improve the quality of as many lives as possible, then it follows that we should maximise the efficiency of healthcare work.
Medical professionals are a considerable bottleneck: they are time-consuming to train and expensive to employ, and the level of specialisation required inherently means that they are not particularly fungible; one cannot typically draw from a pool of one type of medical specialist to fill a labour gap in a different specialty during a shortage.
As a result, workloads are often high in medical settings and the healthcare industry at large, both in terms of individual case load and the related administrative requirements for each individual case.
There are several schools of thought on how to improve this situation. One of them proposes replacing human labour with generative machine learning software. So far, this has had some considerable downsides, none of which appear to be easily solvable.
Given this mixed-to-negative outlook, you'd think that scientists would be united in pushing back, demonstrating how bad of an idea the use of this kind of medical 'A.I' would be. To an extent, this is the case!
Unfortunately, a small but significant chunk of the scientific community is composed of the most gullible thought-wranglers to ever successfully plug in a keyboard, and they're rather undermining the group project.
This is Not Alarmism
While I have a tendency towards hyperbole, the state of affairs is bad enough that its use here would actually soften the blows. I am being very matter-of-fact when I say that the use of machine learning (generative or otherwise) in healthcare is already reducing people's quality of life. And also killing them.
For instance: UnitedHealthcare (the largest health insurer in the U.S.A) denies claims at double the industry average rate (32% vs an average of 16%).
While the company stresses that denying claims is not denying care (as patients can still pay for care themselves), the reality of living in a country where simple procedures can cost tens of thousands of dollars means that denying payment for care is denying care, and it kills people.
Since 2019, UnitedHealthcare has used a machine learning model called nH Predict (produced by NaviHealth, a company that UnitedHealthcare acquired) to estimate the level and duration of care that its vulnerable Medicare Advantage patients will need while being treated for acute illness or injury.
Despite these estimations not always taking into account fairly key factors for health prediction (such as comorbidities or the development of additional illnesses), and despite the fact that nH Predict is only a piece of predictive software and not a human being, its estimations are treated as expert decisions with medical backing when it comes to denying medical insurance claims.
Legally, the process of review for Medicare Advantage (part of the government-enforced healthcare system) is tightly regulated, but the actual process of nH Predict falls short, likely not considering the required factors or weighting them appropriately. Inquiries into its workings are denied due to its 'proprietary' nature.
“[M]any providers said their attempts to get explanations are met with blank stares and refusals to share more information. The black box of the AI has become a blanket excuse for denials."
—Mike Reddy, writing for STAT, in the article Denied by AI.
In 2023, UnitedHealth aimed to keep the rehab stays of all its patients insured under Medicare Advantage to within 1% of the software's predictions.
This 1% target was applied regardless of whether additional care was necessary and justified under Medicare Advantage's rules.
While UnitedHealth insists that "the NaviHealth predict tool is not used to make coverage determinations", that is a lie. It is. Its estimations are used as a target. Staff are instructed to 'defend' denials for coverage by relying on factors stated in nH Predict's outputs. It is, intentionally, making coverage determinations.
In addition to merely being a piece of predictive software, nH Predict appears to have a false positive rate of 90% for its denials. To be precise, when nH Predict's denials are reviewed, internally or through legal avenues, 90% of its denials are found to be wrong and reversed.
A 90% error rate on review is blatantly not sufficient unless the point is to deny as many claims as possible. Which it is:
“A former unnamed case manager told STAT that a supervisor directed her to immediately restart a case review process for any patient who won an appeal. "And 99.9 percent of the time, we're going to turn right back around and issue another [denial]," the former case manager said. "Well, you won, but OK, what'd that get you? Three or four days? You’re going to get another [denial] on your next review, because they want you out.""
—Beth Mole, writing for ArsTechnica, in the article UnitedHealth uses AI model with 90% error rate to deny care, lawsuit alleges.
It may be of note that Brian Thompson, the CEO of UnitedHealth Group (who, again, deny more claims than any other U.S.A health insurer by percentage and by volume), was specifically targeted and shot dead in the street New York very recently. This is not, to say the least, an indicator of high levels of customer satisfaction with algorithmic denial of care.
UnitedHealth is not alone: Cigna, in another high-profile instance of algorithmic denial of care, has "built a system that allows its doctors to instantly reject a claim on medical grounds without opening the patient file", which appears to be breathtakingly illegal.
However, because their software provides summaries, 'flags mismatches', and suggests actions to Cigna doctors based on data in the patient files, Cigna appears to feel its legal obligations to 'review' those files have been met.
This is, it claims, 'standard industry practice', an assurance which mostly confirms that all health insurance companies (and possibly some of the people involved) need to be gutted and replaced with something more fit for purpose.
This is Not an Outlier
The software currently deployed in health insurance is was never going to be 'accurate' in terms of its error rates. Health insurance is simply the business of figuring out how many people it is most profitable to not just let die but actively kill (deliberately and repeatedly interfering with the expected course of healthcare provision is an action, not an inaction).
It is here that I should clarify some terms. The field of 'machine learning' is vast and decades old: it contains everything from simple decision trees to complicated 'neural networks'. Here, we are talking about the very complex kind.
For our purposes, there are three kinds of complex machine learning programs: classifiers, transformers, and generators. Classifiers are the oldest and often more reliable kind: they take data and classify it. Transformers take data and translate it into other forms.
Generators (which are what 'A.I' usually refers to in the current discourse) produce new data. They are the most hyped and least reliable (for reasons we'll touch on later).
The use of machine learning in areas of healthcare with less predatory motivations may seem safer than its use in insurance. It has, after all, seen remarkable success at narrow-focus tasks like folding proteins, mass-sorting research data, and improving statistical analysis (sometimes being more powerful than regression-based approaches).
After all, if doctors are directly trying to save and improve lives, then any tool that helps is good, right? There is even an argument to be made that as soon as software achieves an error rate lower than the (qualified) human average in a task, it is suitable for use.
Unfortunately, partly due to the broad scope of many newly proposed applications and the increasing prevalence of generative machine learning, this is insufficient. Errors, like medical staff, are not fungible; they cannot easily be compared or held as equivalent.
For one, software cannot self-review or be held accountable, reviewed, trained, or reasoned with in the same way or precision as a human can.
For two, the types, severities, and causes of the errors made by machine learning software (particularly generative models) are very different to those made by humans.
For three, the scope of software's deployment provides a far wider potential scale of impact for its errors than for those of humans.
In all three dimensions, errors made by software can be worse, and have worse implications, than those of humans. As we will see, in many proposed 'A.I' use cases these errors would not even replace human errors but instead 'stack' on top of them.
Before we launch into a (long and extended) dig into the proposals and literature, let us consider some hypothetical issues with expanding machine learning software's use in medicine. As a fun little game, you can keep an eye out to see how much of the literature we encounter addresses any of these potential issues, even in passing.
After an error is made, it must be understood and prevented from reoccurring. If a systemic pattern of neglect is found due to a hospital-wide AI system owned by an outside vendor, how much harder is it to address than the neglect of a single doctor, or even that of an entire department?
Where does the responsibility lie, particularly regarding compensation and redress? Are the lower-seniority staff who acted upon the software's conclusions more or less culpable than if they'd acted on behalf of human instructions?
When analysing human mistakes, clear patterns emerge in terms of overwork and stress, allowing colleagues to often anticipate periods where their tired or stressed co-workers may make more mistakes and compensate accordingly. If no such pattern is present for software (if error rates are functionally random), will this increase rates of errors that are never caught or caught too late?
As a primary purpose of introducing machine learning tools is to reduce the burden of human labour, how much oversight (to screen for unpredictable, confidently-presented errors) will users actually perform? What sort of cognitive load does editing and error screening carry compared to producing accurate work oneself, and how sustainable is it?
A critical flaw in any piece of sufficiently opaque machine learning software may be totally unpredictable, have absolutely no diagnostic indicators, and plausibly reoccur in any future version of that software or equivalent product. How should this be prepared for?
There, that should be enough questions to chew on for now.
Complex machine learning software is not intrinsically 'bad' on some moral level. It is, however, materially different from more traditional software, which is less complex and does not rely on an inscrutable 'black box' of statistical associations. It is less auditable, less predictable, and harder to trust than something like a database.
Nonetheless, too many people are still proposing that complex machine learning software, and specifically generative software, is perfectly suited for use in medicine.
When it comes to medical technology, it is important to minimize risk and unpredictability. You would likely not appreciate your doctor using a database or word processor if it unpredictably changed even 0.5% of keystrokes when entering data.
Error rates as low as 0.012% in laboratory sample tests are considered a serious issue in need of addressing due to patient impacts. Why, then, does so much of the literature become far less risk-averse when machine learning is involved?
Why are error rates of 5% per diagnostic task seen as acceptable for some implementations? Why is there a common explicit assumption that generative machine learning technology is going to linearly and rapidly improve over time, not just in raw power but in terms of the scope of tasks it can complete?
To paraphrase a YouTube comment I saw over a year ago that has stuck in my brain, far too many scientists writing on complex machine learning seem to interpret a 'balanced analysis' as 'all arguments are treated as equally plausible regardless of evidence'.
Much like how errors are not fungible, inspections of an idea's 'pros and cons' should not simply treat each individual 'pro' or 'con' as a tally mark on a scoreboard, all equivalent in importance; analysis should be more complex than seeing which side is supported by a bigger number of abstracted 'reasons'.
This is Not Just Theoretical
There are lots of proposed uses for complex and generative machine learning in medicine, but the best way to illustrate some key problems is to start with a real example.
In one recent case, a commercial machine learning model was (after more than a year of preparation) deployed at a specific institution without direct supervision to make low-risk triage diagnoses of radiographs of patients with knee issues. On the very first day of its clinical implementation, it made a very straightforward mistake for "no obvious reasons".
During independent testing, the tool had achieved an error rate exactly comparable with that of the diagnoses of human radiologists regarding osteoarthritis classification. This was deemed acceptable.
Unfortunately, after this first error, it became clear to staff at that institution that the unpredictability and unsolvability of the software's errors and the unknowability of the root cause of each individual error had an impact on people's ability (and willingness) to work alongside and trust the software.
The key problem, of course, is that a human radiologist is plainly able to state and discuss their rationale behind a decision to their colleagues; not so for the software.
Logistically, without even considering error severity, any opaque and truly random software errors are more dangerous and harder to work with than human errors, even at the same rate of occurence.
Looking at radiographs and triaging the likely stage of osteoarthritis is a very narrow task with straightforward paramaters. Perhaps additional layers of review will make this more reliable?
Unfortunately, there are roadblocks here too. While it is often suggested that additional layers of machine learning software can be used to review the outputs of automated tasks, or perhaps that tasks can be performed several times and internally compared to improve reliability, the reality falls short. Computing costs become prohibitive and improvements are minimal.
Instead, the use of a generative Large Language Model to provide 'reasoning' or 'explanations' for the results of a machine learning process is offered up instead. This falls prey to a core issue: they are language models, not knowledge models, no matter how much computing power they are given or data they are fed. They are not built to reason and will often simply produce correct-seeming but erroneous outputs.
This may prove to be a fundamental issue, even with LLMs specifically designed to provide extensive reasoning for their own decisions or the decisions of other programs they are monitoring.
“The reasoning errors carried out by the models suggest that even the explanations need to be evaluated in-depth. The amount of memorization and discourse flaws output by these models invite for a deeper reflection[...] This can be the subject of further work: many models generated consistently grammatical text, but with errors in both formal and informal reasoning[...] We can see that these LLMs do not consistently withstand a critical evaluation of their output, and their argumentative and reasoning capabilities are lacking."
—Conclusions of the 2023 paper An evaluation on large language model outputs: Discourse and memorization.
It may, then, seem obvious that the only way to safely use these time-saving but unpredictable programs is to place humans firmly in charge and simply ensure they are 'assisted' by the software, reviewing its every output.
Counter-intuitively, this can backfire when using complex analysis software, resulting in higher error rates, not lower ones.
This is because humans may discount a significant amount of a program's output in an instance where even a small part of its output has some uncertainty, leading to helpful data being disregarded and thus worse outcomes.
This mistrust is not always rational, but it is understandable. The general feasibility of 'replacing human analysis with human review' is further complicated by the fact that reviewing an analysis provided to you is a non-trivial congnitive skill, and is not actually self-evidently 'easier' than producing your own analysis.
The problem is worsened yet further by the fact that generative machine learning software, by its nature, can be harder to error-check than a human: it invariably produces good and bad data with equal confidence and persuasiveness, and it may be hard to know where your human revisions will help or hinder.
In one study, while the assistance of GPT-4 increased 'creative product innovation' (coming up with speculative ideas), its actual use in real business problem solving scenarios "resulted in performance that was 23% lower than that of the control group".
Perhaps frustratingly, those who were primed and warned about GPT-4's tendency to provide false answers did worse still (29% worse than the control group) than those who simply used the LLM without being warned (16% worse than the control).
As such, it can be very hard to safely and successfully integrate complex machine learning analysis into any real-world application, particularly medicine. The issues of software variability and human nature don't cancel out: they overlap.
These Errors Are Really Not Comparable
So, complex machine learning software, particularly of the generative kind, isn't often suited for applications in daily medical practice: it is hard to trust and inspect, and even comparatively low error rates don't offset the unpredictability of its errors.
We should talk some more about comparing risk, though. When talking about the use of machine learning software in healthcare, flat 'error rates' are often used as a final arbitrator of quality.
In terms of simple overall rates, rough estimates for overall human medical error are, well, rough, but appear to be around 5% to 10% overall, with a 0.3% rate of serious issues resulting.
Closer review indicates that there are very low rates of treatment-affecting errors in specialist care tasks. For example: in a review of 3,251 biopsies, 87 errors of any kind were found, 15 of which definitively affected the quality of care. Of another sample of 4,192 biopsies, 146 errors were found, 32 of which were under-estimations of severity.
Drawing back to a wider view, the '5% to 10%' rough estimate of overall human error is made less daunting by findings that, of confirmed errors (analysed in cancer diagnosis), the majority typically resulted in no harm or were noticed and prevented before harm; of instances of harm, the vast majority caused limited harm, meaning that further or repeat non-invasive tests were needed, accurate diagnosis was slightly delayed, or minor and brief 'morbidity' was caused and resolved.
I present this summary not to say 'humans are perfect', because clearly this is not true: Things can and should be improved. I'm just aiming to provide reasonable context for discussing types of errors and error rates.
One of the issues at play is that error rates for humans are often given on a per-patient or per-case basis, encompassing all individual tasks taken in their care. For the literature on machine learning, even for models that can perform multiple tasks, error rates are typically assessed (and presented) on a per-task basis.
This is part of why I contrasted BASE jumping with elevator maintenance issues in this article's opening: if you assess the rate of elevators breaking down per instance of use, instead of per day (and compare the types of harm done), it becomes viscerally obvious how disingenuous one can be when comparing unlike statistics.
In other words, a mere 1% error rate for a machine learning chatbot that advises patients on likely diagnoses sounds not terribly alarming at first glance, and might even seem preferable to your local doctor, who makes mistakes with your care far more than 1% of the time...
...until you realise there is specifically a 1% chance that the software in question will make a mistake every single time a patient enters a new query — something that may happen in each individual use case upwards of a dozen times as the patient asks a series of questions, seeking clarifications or expanded explanations.
This is a key part of what so often makes me feel (to be delicate) completely bugfucked when reading the literature on medical machine learning.
If there is a 0.5% chance of a sub-task failing, but that sub-task takes place several hundred times a day, you have: a problem. Not a 'promising approach'.
Things get worse, however, because so far I have been kind to machine learning. I've briefly mentioned that generative models have their own reliability issues, but now it's time to get specific.
Generative models, and large language models more specifically, have captivated the collective imagination. People are trying to cram them in everywhere. This is a problem because that 'per task' error problem is worsened by a largely unique flaw: hallucination errors on even the simplest of tasks.
Unpredictably, a non-trivial amount of the time, they will make things up. This is fundamental to how they function. This is an issue specialist researchers consider to be unsolvable.
“[Even in vastly simplified formal conditions], we present a fundamental result that hallucination is inevitable for any computable LLM, regardless of model architecture, learning algorithms, prompting techniques, or training data[...] We emphasize that since hallucination is inevitable, rigorous study of the safety of LLMs is critical."
—Conclusions of the 2024 study Hallucination is Inevitable: An Innate Limitation of Large Language Models.
ChatGPT, to take a well-known large language model, can produce non-trivial errors from a single prompt involving simple knowledge that is well-represented within its dataset (spelling a simple word, counting instances of letters, or assessing the truth of extremely simple logical statements).
In a 2023 hallucination test, ChatGPT was found to hallucinate in factual responses 19.5% of the time, and was only able to fact-check hallucinations in provided LLM-generated text 79.44% of the time, with accuracy dropping to 58.53% when checking for hallucinations in summaries specifically.
Even when augmented with up-to-date training data, web access, and error verification components (in something close to a best-case scenario), ChatGPT's responses are still often either uncited or incorrectly cited.
"However, even in the GPT-4 RAG model, we find that up to 30% of statements made are not supported by any sources provided, with nearly half of responses containing at least one unsupported statement[...] one response by GPT-4 RAG indicated that criteria for gambling addictions are equally applicable across all individuals and groups. But the source it referenced concluded the opposite[...] In another example, the model recommended a starting dose of 360 joules for a monophasic defibrillator (one where the current runs one way to treat a patient with cardiac arrest), but the source only mentioned biphasic defibrillators (where current runs both ways). That failure to distinguish can matter greatly."
—An explanation of pre-print results in the Stanford University article Generating Medical Errors: GenAI and Erroneous Medical References.
This is clearly not up to standard for real-world use, right?
In absolutely unfairly generous conditions, when summarising specific non-specialist texts of relatively short length (not aggregating data based on queries), optimised LLM error rates generally sit between 2–4% per task. There is likely no way to reduce this chance to zero.
Any time you see references to 'human review' or 'users should exercise caution', remember that it is often the prompted task of an LLM to produce rhetorically convincing, true-seeming text to time-poor people who are asking for assistance in resolving uncertainty.
This should make it clear how insufficient it is to suggest that users of LLMs for factual applications should simply exercise caution and carefully check all generated outputs.
It is not clear enough for some, however, because despite all of these very clear risks to patient health, parts of the scientific community keep on recommending the low-oversight use of generative machine learning software.
This is Not How You Should Cite Your Claims
The editorial paper titled ChatGPT and other artificial intelligence applications speed up scientific writing provides a clear illustration of one side of the quality divide in the scientific literature on the potential applications of machine learning technology.
Broadly, the paper is a short introductory piece aimed at people who write medical and scientific literature and have a passing familiarity with ChatGPT. The paper advocates for the widespread use of generative machine learning technology in the realm of scientific and medical writing. It talks positively on the technology's capabilities and advantages. Partway through the paper, the author writes the following:
"Dear readers, if you have read the previous paragraphs and found them comprehensible, you are entering the world of AI."
—2023 paper titled ChatGPT and other artificial intelligence applications speed up scientific writing.
This is perhaps intended to surprise the reader, prompting them to re-read the previous paragraphs (all of which were produced or edited in some way by machine learning) and reconsider any scepticism they may have had about the capabilities of advanced ML-driven generation, editing, and translation technology.
Unfortunately, this does not have the intended effect, because the paper is of extremely low quality, rife with basic grammar errors, and (if we're being generous) employs a lightweight 'pros and cons' approach that does not consider the actual effects of any of the downsides.
I have been really quite even-keeled for the last 6,000 words, but I really can't stand being polite anymore. This paper is shit. It's ten kinds of shit in a five-shit-capacity bag. The author writes perfectly decent papers on medical matters, but here they have simply decided to piss away all vestiges of integrity and rationality because Cool Words Machine Go Brrrrrrrrrr.
In relying on generative tools for all stages of this editorial's production, the author has just cocked the whole thing up completely. The paper's only figure is described totally wrong in its caption. In a nonsensical pivot, the paper briefly calls the use of machine learning in paper-writing "clearly unethical", but then continues to recommend its use without reservation.
The author actively recommends that scientists do not need to know anything about machine learning in order to use it to help them write scientific material.
In a flourish I simply could not have made up if I tried, this seven-lane pileup of a paper wraps up by providing this ChatGPT-generated summary: "AI-generated writing is still considered unethical and its accuracy is questionable. But, scientists should embrace AI tools and use them to overcome writer’s block, without having to understand the underlying algorithms."
This paper is, on its face, comically bad. Short enough to read in a few minutes, really, but still quite bad. I would consider it overkill to give it so much focus if it had not, somehow, been published and then positively cited by roughly a dozen other papers to support generative machine learning's usage in the sciences.
One paper cites the article to support the assertions that ChatGPT "speeds up research and analysis, and promotes equity by assisting non-native English-speaking authors" and is "relevant for improving writing, reducing redundancies, suggesting synonyms to enrich vocabulary", concluding that "in the set of articles analyzed, ChatGPT emerges as a valuable tool for researchers and scientists by improving writing, speeding up tasks, enhancing quality and offering support in various areas, boosting the efficiency and quality of academic and scientific production."
Another paper cites the article when claiming that "[generative] AI is already capable of providing definitions, complete document translations, (re)writing, and summarizing". It presents these alleged capabilities without caveats, caution, or balance, instead advocating that clinicians should be using these tools to stay "up to date".
It goes on to posit that "AI can act as a second clinician in the decision-making process, where the human clinical expert can interact with the "artificial clinical expert", leading to more accurate decisions" and that "this human-machine interaction may be particularly valuable for those just starting out their careers".
Cases like this are why I was so thorough above regarding the risks, human–machine cooperation issues, and so forth. How else to appropriately frame the repugnancy of these authors, who are advocating for the widespread use of very newly developed Lies Unpredictably Machines; for inexperienced people trying to research and produce factual information in risk-averse contexts, no less?
I mean: "this human-machine interaction may be particularly valuable for those just starting out their careers", for fuck's sakes. As a reminder:
“All LLMs continue to have clinically significant error rates, including examples of overconfidence and consistent inaccuracies."
—2024 study, Comparative Evaluation of LLMs in Clinical Oncology.
It is not simply that the citation practices of these two papers are very bad throughout (they are), but that this poor source comprehension is acting in service of irresponsibly misleading the reader and proposing ideas that, in the case of the second paper particularly, would unpredictably compromise the safety of those seeking medical care.
The author of ChatGPT and other artificial intelligence applications speed up scientific writing is no more a fan of reading comprehension than the authors citing their work, and makes some extremely funny errors in their citations.
For example, a study cited in his very first paragraph to support the assertion that "discussion of [AI use] in medical journals has lagged behind" is actually an extremely clear report on the dangers of the uncritical use of LLMs in medicine:
"ChatGPT produced a complete [medical referral] letter that included an appropriate heading and formatting and provided a clear explanation of the examination, supported by fabricated references. Current limitations of using ChatGPT for manuscript generation include [...] its tendency to fabricate full citations, and the inability to piece medical knowledge together appropriately. While it can write authoritative-sounding pieces on general radiology, it puts medical knowledge together in a way that often does not make sense on careful review."
—2023 study, ChatGPT and Other Large Language Models Are Double-edged Swords.
The fact that this fairly explicit caution against LLM usage in medicine has been laundered into 'LLMs are very capable in the medical field and we should expand their use immediately' in just two layers of citation is, to be blunt, an act of misconduct so great there should be professional and perhaps legal consequences for everyone involved.
Does Nobody Know How To Fucking Read?
Because of the sheer sloppiness of the prior examples, I'd like to provide a much more in-depth look at a more serious and well-composed paper that, nonetheless, shows the same pattern of issues with citation comprehension.
It is despite a very clear array of risks in the literature (and a fairly clear level of unmitigatable risk in its own findings) that the study A strategy for cost-effective large language model use at health system-scale begins its Introduction section with the following:
"Large language models (LLMs) can process large volumes of text and produce cogent outputs. This ability also extends into realms that are highly specialized with complicated subject matter, and has particular promise within medicine."
—Introduction of A strategy for cost-effective large language model use at health system-scale.
I take no particular offence at the bulk of this study: much of its length is dedicated to figuring out if batch-processing requests is a feasible way to lower the cost of using a commercial LLM. I take issue, however, with just about everything else this study says about LLMs and their capabilities in medicine.
It begins by backing its opening sentences with two citations. One is a study that very blatantly does not highlight any "particular promise" for ML and LLMs within medicine above any other field, instead saying that the technology is currently being used and tested with "mixed results".
The other citation, Opportunities and challenges for ChatGPT and large language models in biomedicine and health, might actually, if you squint, support the technology's 'particular promise' in medicine. In particular, it cites a cluster of five of other studies that have shown strong performance for certain machine learning models. We can treat these five studies, running back up the chain of citation, as the primary support for the claim.
The first sub-citation in our dive is a study that tests a carefully custom-tuned LLM, Flan-PaLM, which performs better than all other models but "inferior to clinicians" on a testing database of medical questions.
Some of the paper's analysis is of a pure percentage result of accurate answers provided to multiple-choice questions (with results between 57%–79%, depending on the test). The further analysis provided on open-answer questions is not encouraging:
“Our human evaluation clearly suggests these models are not at clinician expert level on many clinically important axes."
—2022 Google/Deep Mind study, Large Language Models Encode Clinical Knowledge.
It concludes even less enthusiastically:
"The advent of foundation AI models and large language models present a significant opportunity to rethink the development of medical AI and make it easier, safer and more equitable to use. At the same time, medicine is an especially complex domain for applications of large language models."
—2022 Google/Deep Mind study, Large Language Models Encode Clinical Knowledge.
This was possibly the most polite and diplomatic way for Google (heavily invested in AI hype) to have said 'at present, none of this will work in medicine and a completely different approach is needed. We don't expect much serious progress in this area.' Not exactly showing 'particular promise', so far.
The second sub-citation is Training language models to follow instructions with human feedback, a study that demonstrates a proof of concept for aligning the goals and truthfulness of LLMs, but nonetheless showed no improvement in bias, small improvements in toxicity, and significant remaining vulnerabilities to falsehoods or mistakes in user prompts, hallucinations, and even simple factual errors.
"To give a few examples: (1) when given an instruction with a false premise, the model sometimes incorrectly assumes the premise is true, (2) the model can overly hedge; when given a simple question, it can sometimes say that there is no one answer to the question and give multiple possible answers, even when there is one fairly clear answer from the context, and (3) the model’s performance degrades when instructions contain multiple explicit constraints (e.g. “list 10 movies made in the 1930’s set in France”) or when constraints can be challenging for language models (e.g. writing a summary in a specified number of sentences)."
—2022 OpenAI study, Training language models to follow instructions with human feedback.
The third citation is simply a link to an OpenAI blog post about 'OpenAI Codex'. It does not contain any relevant information on medical or biomedical usage; it does contain a brief overview of the company's LLMs and their ability to undertake natural language processing tasks. Perfectly reasonable as background information, but no indications of 'particular promise' in medicine.
The fourth sub-citation in the cluster is Capabilities of GPT-4 on Medical Challenge Problems, which presents results showing GPT-4 outperforming the previous 'state-of-the-art' model, Flan-PaLM (discussed above) on multiple choice tests by between 1%–17%, depending on the test. At every stage, the paper also makes mention of the serious, currently unavoidable risks inherent in LLM usage, despite this improved performance, including a particularly eloquent and emphatic explanation in the Results:
"Significant risks with uses of large language models include inaccurate recommendations about rankings (e.g., with differential diagnoses) and sequencing (e.g., information gathering, testing), as well as blatant factual errors, particularly with important omissions and with erroneous generations, often referred to as hallucinations. LLM hallucinations can be particularly difficult to detect given the high linguistic fluency of the models and the ability to interleave inaccurate and ungrounded assertions with accurate generations. Such hallucinations can include incorrect or misleading medical information which necessitates careful review and fact checking. Thus, extreme caution is required when using LLMs in high-stakes medical applications, where incorrect or incomplete information could have serious consequences for patient care."
—A very reasonable study, Capabilities of GPT-4 on Medical Challenge Problems.
The fifth citation is Towards Expert-Level Medical Question Answering with Large Language Models, a study that again iterates on the results of previous work and tests an updated version of Flan-PaLM, Flan-PaLM 2, on multiple-choice medical tests.
Importantly, however, it also tested written responses from Flan-PaLM 2, compared them with written responses from human physicians, and found that there was a subjective preference for the LLM responses among a sample of fifteen physicians (from the US, UK, and India) and six lay-people (from India only).
Flan-PaLM 2's answers were much longer than physicians' answers and were rated by lay-people as more helpful overall, which is definitely indicative of the LLM producing writing that more appealed to lay-people. However, physicians did not rate the LLM responses as favourably, and no in-depth analysis of the factual quality of the LLM's answers and errors is provided in the paper.
It is also notable that while the physicians were from a range of countries (and the testing conducted in English), the lay-person raters were all based in India, and there were only six of them.
Despite optimism, the paper comments that LLM answers are still "progress[ing] towards physician-level performance", in no small part due to their persistent tendency to produce answers "innapropriate for use in the safety-critical medical domain" in a way that human professionals do not.
If we look over these five sub-citations, we can see they primarily provide evidence that the field of 'LLMs addressing test-format medical questions' is progressing. However, that is the general limit of the optimism, with a significant amount of each paper dedicated to discussing risks and issues that have no current solution, nor a firm path to one.
Thus, we are provided a clear example of our core paper (A strategy for cost-effective large language model use at health system-scale) laundering citations to make the leap from 'showing limited development with critical weaknesses' to 'particularly promising in this area'.
As a single claim, this is irresponsible but not hugely damning, but the paper then goes on to, quite egregiously, fail to engage with the downsides of nearly every single one of its cited references, in some cases quite drastically misrepresenting their conclusions, in order to advocate for the use of LLMs in healthcare settings.
For example: still in the Introduction, it is said, without any further context, that LLMs "can perform" three specific, narrow-focus tasks based on electronic health record data (with one citation per task):
- Extracting important contextual social information from free-text notes on patients and categorising it into specific codes;
- Classifying annotated pathology reports to create a fully-annotated database;
- Processing and interpreting electronic health records as a whole.
The study cited for extracting information from written medical notes tests the ability of a number of LLMs to extract six specific social factors: "employment status, housing issues, transportation issues, parental status, and social support."
While success rates for the best-performing models were above 90% overall, all models retained a persistent vulnerability to changing their results when race or gender was varied in otherwise-identical records (i.e., contextual bias).
The application of this narrow-scope data extraction is not that the task itself is particularly challenging or even time-consuming for individual doctors (who have already written the long-form notes with the relevant information), but that Social Determinants of Health are rarely extracted and formally recorded in healthcare settings as a whole.
The study cited for analysing reports tested several LLMs on interpreting and classifying breast cancer pathology data, finding that the best-performing LLM had an average success rate of 86%, with one outlying factor being detected correctly 98% of the time, most sitting between 80%–88%, and three others below 80%, with one being correctly detected as little as 70% of the time.
Specific and recurrent mistakes included the false inclusion of grades of tumour where none was stated in the report, common issues with defining the size of tumours, and either missing that benign sites were examined or miss-classifying them as tumours.
For the third example, processing and interpreting whole electronic health records, the cited study showed a new, custom-trained model improved on existing models by single-digit percentage points in the task of recognising specific information present in health records, with results between 88%–90%.
However, in tasks related to further interpretation and question answering, the state-of-the-art model performed considerably worse, at 74%. While these are undoubtedly impressive technical accomplishments, the current clinical applications of 'a model that is three-quarters accurate at answering questions about static data and can usually figure out if specific factors are present' are limited.
Of the three tasks that our core paper says 'can be performed' by LLMs, this is currently clearly untrue for two tasks, for which LLMs produce serious errors at rates flagrantly unacceptable in real-world medical treatment or research applications.
Saying LLMs 'can perform' these tasks is a little like saying first-year med science students 'can perform' medical tasks: yes, sometimes, but we're not all gung-ho to skip the next three-to-five years of study and shove them into clinical practice, are we?
To be as fair as I can, there is an arguable limited use case for the third task; while having less accuracy than a human and retaining persistent biases that produce errors, this trained LLM may be acceptable for near-future use in the mass annotation/coding of these specific social factors in patient records, solely in research applications and with human review, due to its speed at extracting a narrow scope of information to be specifically, separately encoded. Nonetheless, a potential 10% error rate in a relatively straightforward data processing task is not ideal.
All three of these studies include clear caveats and risk assessments. Neither these, nor their implications, are mentioned even in passing in our core paper. This trend continues, with the phrase 'has been used' persistently being deployed to frame LLMs as fully able to do tasks, maybe even in real-world use cases. In each case, the reality is rather that they have simply been tested for use in these areas — always with mixed results. In rough order of appearance:
An LLM that our core paper says has been "trained to serve as [an] all-purpose prediction engine for outcomes" was trained on a limited set of five prediction tasks and still consistently had error rates as high as 20% in two of the tasks. It does not appear to serve an 'all purpose' function, and has not been deployed in any real-world setting.
Despite being framed as such, LLMs have not "been used" for the prediction of clinical acuity or emergency room admissions; the cited studies indicate they have been tested for such applications, with roughly 80% correct prediction rates, under-performing human estimations and showing no clear path to usage in these real-world environments.
Two studies, cited to indicate LLMs can provide better-quality healthcare data summaries to patients than doctors, instead (when read beyond their titles) provide fairly obvious examples of how patients can be led to prefer the confident writing of LLMs — a confidence which can conceal their unavoidable errors:
“The ensuing safety analysis highlights challenges faced by both LLMs and medical experts, as we connect errors to potential medical harm and categorize types of fabricated information."
—2024 study, Adapted large language models can outperform medical experts in clinical text summarization.
“Eighteen reviews (of one hundred examples) noted safety concerns, mostly involving omissions, but also several inaccurate statements (termed hallucinations). Implementation will require improvements in accuracy, completeness, and safety."
—2024 study, Generative Artificial Intelligence to Transform Inpatient Discharge Summaries to Patient-Friendly Language and Format.
It should be noted that despite the presence of these unavoidable safety concerns and factual errors, both of the above cites do make efforts to downplay the risks and ultimately advocate for the use of LLMs to write summaries for patients. If roughly one-fifth of your outputs have "safety concerns" then it is not a good system! It is not a safe system! I feel like I'm going fucking mad! Did you read your own data or not?
Another claim, that LLMs "can effectively answer patient messages", cited a study that only analysed a non-real-world test of an LLM's ability to generate patient messages. It did badly.
“It was felt by the assessing physicians that the LLM drafts posed a risk of severe harm in 11 (7.1%) of 156 survey responses, and death in one (0.6%) survey response. The majority of harmful responses were due to incorrectly determining or conveying the acuity of the scenario and recommended action[...] Compared with [human] responses, LLM drafts were less likely to include content on direct clinical action, including instructing patients to present urgently or non-urgently for evaluation, and to describe an action the clinician will take in response to the question[.]"
—2024 study, The effect of using a large language model to respond to patient messages.
In this study's conclusion, compared to the text that is optimistic about LLM usage, four times as much text is dedicated to reiterating the critical downsides and risks of LLM usage to even draft patient messages, let alone produce final versions to be sent. It ends with a call for all vendors of commercial patient-facing machine learning software to provide more transparency about their models as evaluations are "urgently needed".
Again: our core study under analysis cited this study to support that LLMs can "effectively answer patient messages".
It is here, though, that our core study runs out of steam. After a slew of fifteen very bad citations in a row, it engages in some idle speculation, and then moves on to its core purpose: being concerned about the cost efficiency of using commercial LLMs.
This is, in itself, not a sin, but the way this study frames the industry as being at the point where it is reasonable to worry about optimising the cost of API calls of LLMs being used to answer medical queries is... maliciously misleading. That it does this by actively downplaying the risks and issues in well-written studies (to the point of outright misrepresenting the conclusions of the papers it cites) is egregious.
The fact that it tested the baseline error rates of a subset of LLMs and considers an error rate of "less than 5%" to be hardly worth a comment (given the context of the proposed use in healthcare) is merely disappointing in comparison.
It is of note that 30% of articles dealing with ChatGPT's usage in medicine do not substantially "note inaccuracies" regarding its output, suggesting a very serious blind spot in the field.
I do not expect all studies on machine learning to be in-depth explorations of the technology's key flaws and risks. I do, however, expect all studies actively exploring potential real-world use cases to take these risks seriously, or at least properly acknowledge them and address their impact on the proposed use case. Not 'mention they exist in passing', but 'mention how, specifically, these risks impact this specific use case'.
This is, quite often, apparently too high of a bar to clear.
It's Bad Research, All The Way Down
I wish, I really wish, that there wasn't so much material to work with. Sloppiness of this kind is riddled throughout the scientific literature on medical 'A.I', and it's taken this long to cover a relatively small amount of studies.
Here, as I rattle through an overview of some other highlights I've found, I'll have to trust that the above ten thousand words of more detailed grinchery has demonstrated I'm not exaggerating when I say things like 'this study is materially harmful' or 'a clown-car full of amatuer clownology students could produce a better paper than this'.
I'd also like to be clear that I read a lot of papers. My work exposes me to an effectively random subset of them. In wading through the literature on medical machine learning, I have seen a far higher rate of muck, and muck of a kind that I don't usually see.
We'll start with a 2019 overview of AI in medicine that provides a strikingly funny example of the kind of empty 'vaguely listing pros and cons' approach, providing as an illustration of its points the kind of content-free figure that one might find in a high-school PowerPoint presentation:
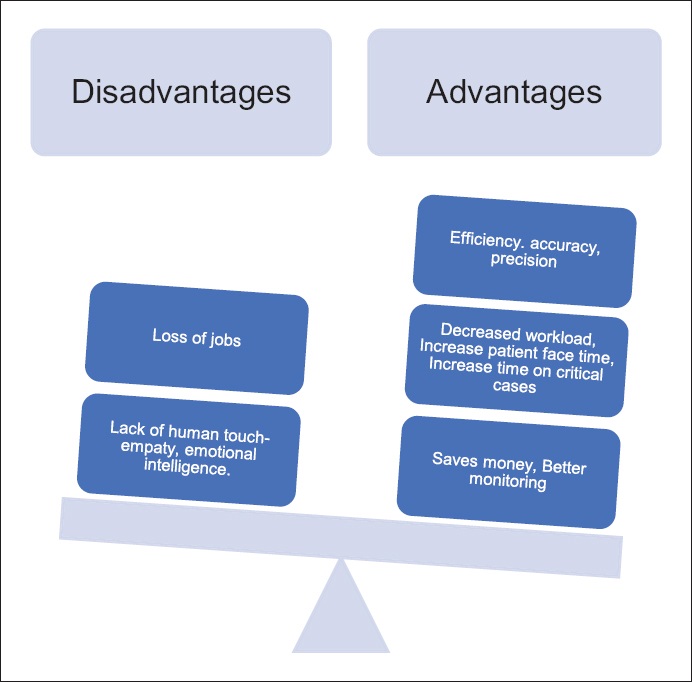
Reasonably sure this graphic was actually made in PowerPoint.
Despite a lack of actual analysis and an uncritical acceptance of the (now falsified) idea that machine learning will simply continue to improve in accuracy with no fundamental limits (the authors are not specialised in information technology), this study has been cited over two hundred times since publication.
This is frustrating, because well-written and conclusive articles that have negative conclusions (such as Let's Have a Chat: How Well Does an Artificial Intelligence Chatbot Answer Clinical Infectious Diseases Pharmacotherapy Questions?) don't have anywhere near that level of reach. Actually, that example appears to never have been cited at all.
An article I briefly mentioned in a prior section advocates for the increased use of generative machine learning on the basis that it will improve "evidence-based practice" in medicine. Ironically, it does so while not adhering to evidence-based practices in scientific writing. It also pulls the 'abstracted pros and cons slightly weighted towards the pros side' move:
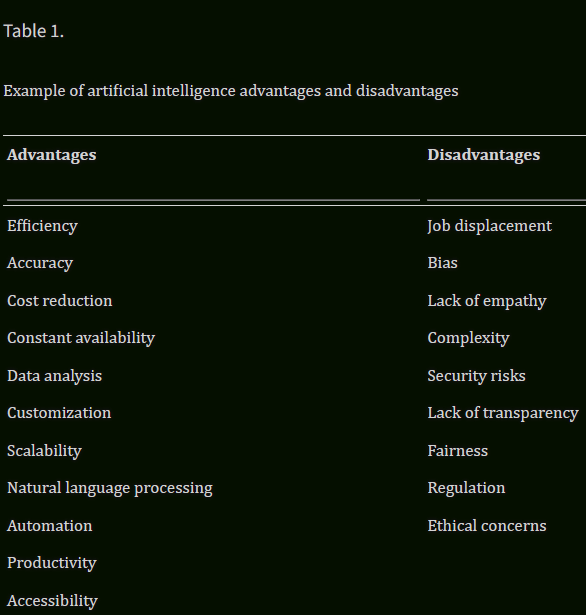
Analysis is when you list things and there are more of one type of thing than the other type. That's what analysis is.
I would not consider that a particularly coherent summary of machine learning's potential use in medicine, to be honest. The paper downplays the persistence and unsolvability of information hallucinations in LLMs as a 'bug' that means anyone using it will just need to "do their own homework". It does not elaborate further.
In one section, it does cite up to ten separate studies per claim when making claims about machine learning's ability to perform tasks: it, however, makes the exact same mistake of repeatedly conflating "performed okay in a limited trial" with "ready for deployment in high-risk areas of medicine".
Much idea laundering is done when studies frame the 'background' of an area of study in their introductions. This is also where a lot of quite funny misconduct comes into play.
One particular study says there is "increasing recognition of ChatGPT’s benefits in medical writing" and then cites four separate papers, three of which are so heavily leaning against the use of LLMs in medicine that their titles alone indicate they are not 'increasingly recognising the benefits': one straightforwardly titles itself "a cautionary note".
Undaunted and clearly quite proud of their inability to process basic information, the authors of this remarkably bad assessment of LLM use cases make the explicit claim that simply rewording key words in a paragraph (via ChatGPT, naturally) is sufficient to "prevent plagiarism" in one's writing.
For instance, consider this source text:
“Spondyloarthritis (SpA) is a diverse collection of chronic rheumatic diseases characterized by inflammation in the spine and sacroiliac joints. This group includes a prevalent form known as radiographic SpA, which primarily affects the axial skeleton."
The authors declare that using the following lazily tweaked version is not plagiarism:
“Spondyloarthritis (SpA) represents a varied group of persistent rheumatic conditions that primarily cause inflammation in the spine and sacroiliac joints. A common variant of this group is radiographic SpA, which chiefly targets the axial skeleton."
Genuinely, this just an endorsement of using LLMs to plagiarise other people's work. Taking someone's words and slightly rephrasing them to use as your own is textbook plagiarism, not 'plagiarism prevention'! The lead author keeps writing papers on 'using AI in medicine' and, somehow, nobody has taken their keyboard away yet.
It was while reviewing these papers (unremarkably bad, by the way) that I was struck by the general ongoing refusal to simply say 'these generative models are not acceptable for use in medicine'. It baffles me.
Even otherwise well-balanced and critical studies, including reviews of other critical research, simply clunge on about how medical staff 'must be aware of the limitations' and that the monumental risks and drawbacks simply "underscore the importance of combining artificial intelligence tools with professional expertise and critical thinking".
One study which included an example of two successes out of a set of twelve tests concluded cheerfully that "ongoing advancements in AI technology offer prospects for improved performance and expanded applications in the medical field, warranting continued research".
Another study suggests that an 88% success rate at identifying just plain old normal red blood cells (and a 50/50 rate of identifying abnormal red blood cells) is "excellent for identifying red blood cell morphology, particularly inclusion bodies [and] can be used as an auxiliary tool for clinical diagnosis", which feels like overstating the matter a little.
On some level, I understand that 'further research is warranted' is a boilerplate saying, almost a pure reflex. On the other hand, sometimes you just have to say that something doesn't fucking work and suggest other options. That's normal. It's so easy. Please. Please do it. Pick something else to try. Please.
Unfortunately, people are just too excited about the Words Machine What Makes Words, so instead we just have a deluge of bad science from people who, by all impressions, struggle to do simple technological tasks like 'access the settings on their phone' or 'remember where they saved their files on the computer'.
For instance, here we have a review of potential LLM usage in dentistry that excitedly advocates for LLM use in diagnosis, treatment planning, and patient management without substantially engaging with the risks or their implications beyond the logistics of implementation and data management.
An alleged 'analysis' of the ethics involved in medical LLM usage practically fellates the concept, mentioning the generic 'big data' issues of patient privacy, bias in data sources, and overall cybersecurity, sweeping aside any potential for erroneous responses by saying LLMs are simply "highly efficient but lack clarity in their decision-making processes" without any elaboration.
A single mention of the hallucination problem is dismissed by saying that health professionals are "well positioned to adapt" to the issue. Given the lack of substance throughout, I am inclined to think there was not-insubstantial undisclosed LLM usage in the writing of this study: so it goes.
An analysis of LLMs in medical education and healthcare plainly states it intends to promote machine learning usage and, for downsides, lightly mentions only the risk of bias or inaccuracies in training data, concluding that "The future of medical education will essentially run with AI-driven technologies". It goes without saying that it really does not evaluate or represent its cited sources very well.
One particularly laughable article claims that 50% of medical jobs will be outdated in 20 years and that machine learning will unilaterally make those who use it far more efficient and effective than those who don't, assuming by default that the field will simply continue to get linearly better and better.
One article confronts the rising problem of 'alert fatigue', as software and various medical alert devices wear down the attention of medical staff with false positives... but then suggests that the use of machine learning, instead of contributing to this (in terms of a human needing to carefully review all generative output for hallucinations, etc), will solve this problem.
This is likely not the case: at the very least, industry adoption of medical machine learning is likely going to result in a further uptick of non-medically provided health advice from the internet at large, increasing the alert fatigue of patients and the doctors who treat and reassure them.
People seeking health information are already facing a deluge of pseudo-informative slurry: there are, for example, more than 100,000 individual mobile phone applications designed for the 'dissemination of health and medical information', with no real quality control at play in the app market at large. Official mass adoption of the Misinformation Vortex to communicate with patients isn't going to make things better.
To tie up, one study that does deserve a closer look is a scoping review for health care stakeholders. It opens badly, with empty sentences like "The development of AI-specific ethical frameworks could facilitate safer and more consistent development of AI tools in health care by preventing the misuse of AI technologies and minimizing the spread of misinformation" (this is one of those sentences that means less the more you examine it).
It then goes on to say "chatbots have the potential to change access to care options for people who live in rural or remote areas and do not have easy access to health care providers in person or through telemedicine", but does not elaborate on how a chatbot, specifically, would be viable in situations where telemedicine is not.
This is followed up with "Chatbots can result in savings for health care providers as well by deferring some patients away from in-person appointments", which is a staggeringly brutal thing to propose without any discussion of the implications for the people who would, you know, be deferred from trying to set up a medical appointment by an automated system?
When claiming chatbots are found to be "effective for supporting healthy behavioural changes", it cites two studies: one review of fifteen trials of chatbot-supported behaviour modification that found, on average, a one-third compliance rate with very mixed outcomes; and one review of six trials of substance use reduction that found only two of the six studies reported any meaningful reduction in use.
It repeats this claim of chatbot 'suitability' for treating mental health, with equally bad citations (one review of 32 studies that showed no long-term effect and worse outcomes than human text chats; one review of 11 trials that showed a short-term effect only; and one review of 12 trials that showed 'weak evidence' for short term symptom improvements and 'no effect' on overall subjective psychological wellbeing; notably, only two of the reviewed studies even attempted to assess the safety of the chatbots).
And for promoting sexual health (one cited review of 31 studies that indicated no effectiveness for improving condom use, antiretroviral usage, and pre-conception safe sex practices, but mild findings for improving attitudes towards HPV vaccination (but not actual vaccination)).
And increasing physical activity (one cited review of 9 studies, showing 'promise' but no actual results in changing fitness, diets, or weight).
This Technology is Being Used Anyway
I really can't stress enough how, in addition to all of the wider and very real concerns about data bias and suitability, patient/user privacy, the opaqueness of guiding and aligning models, the lack of reliable access to the underlying processes and 'rationale' behind complex machine learning software's decisions, the ethics of allowing any software to make substantial medical decisions, and the ongoing inability to prevent user-end abuse of LLMs of any kind (they are absurdly easy to manipulate and 'hack'), there is that one, core, immovable issue at the heart of it all:
A non-insignificant percent of the time, any instance of this generative software is, fundamentally and intrinsically, going to produce a very convincing untruth in its output, no matter what. You cannot optimise this out. You cannot just add more computing power. You cannot reliably use a LLM to review another LLM's outputs. This is an unsolved and likely unsolvable problem, no matter how big these models get, no matter how much data they are fed.
Despite all of the above, complex and poorly tested machine learning applications are seeing use across healthcare, particularly LLMs. While the use of narrow-scope machine learning (particularly non-generative) for data analysis and research is well established, things like ChatGPT showing up in clinical practice of any kind should make you very, very wary.
One area of particularly rapid growth is in transcription, because doctors fucking hate taking notes, apparently. This may appear relatively low stakes compared to LLMs in cancer diagnosos, but the same risks of error remain. Notes, while simple seeming, are important records of medical data. Note-taking errors can be of clinical significance.
One commercial product, 'Freed AI', places itself as a virtual note-taker that records appointments and produces written summary notes for the medical professional involved. It has a very sleek website and a couple of conspicuous 'definitely not advertising' posts on Reddit from 'real people'. It produces appealing text summaries that are easy to read.
Curiously, nowhere does the website mention that, per testing, Freed performs "below the human standard in the category of accuracy", making mistakes like getting names wrong, failing to understand and summarize complex situations, and being unable to infer context and information that was obvious to a human. Which seems important, no?
Freed performed worse than a human in basic accuracy the majority of the time, according to reviewers of its responses, and made mistakes of the kind that humans generally don't: all for shaving off an average of eight minutes of admin time! Wow, the future is really here.
Some forum discussions on Freed's quality note that while using it is definitely a time-saver, the program is difficult to steer and makes quite basic mistakes, such as misattributing ideas from one person (the doctor) to another (the patient) and having difficulty sticking to required formats. Perhaps unsurprisingly, users care a lot more about saving time and not doing 'boring' note-taking than any errors that might slip past.
Other products on the market (Sully, Heidi, DeepScribe, etc.) are roughly on par.
It strikes me that, particularly in one-on-one mental health care, when I see a professional, I am seeing them for their skills and training. Their ability to extract salient details from a larger picture is one of those skills. Note-taking is a skill and a part of the service they provide, during which they are mentally engaging with the facts of the case. It is not simply an unfortunate administrative burden.
The de-skilling of trained professionals is not a good outcome. This is not an 'audio books don't count as reading' type of complaint: this not a shift in the mode of engagement with information but the straightforward replacement of the need to conduct regular information synthesis, which is a very important skill in healthcare of all kinds (which is, as I am fond of saying, the process of troubleshooting the world's most complicated and least rational machine).
Even those who largely think automated scribes are a good idea have specific, non-trivial concerns:
"If future generations of clinicians grow accustomed to AI doing the bulk of diagnostic review and analysis, there is a risk that their own diagnostic skills might not develop as fully. More critically, should they be required to review patient charts manually—due to AI failures—they may find the task daunting, or lack the detailed insight that manual review processes help to cultivate."
—2024 study, Large Language Models and User Trust: Consequence of Self-Referential Learning Loop and the Deskilling of Health Care Professionals.
Unfortunately, in this study, the proposed solution against deskilling is to adopt a "dual focus on harnessing AI capabilities while enhancing unique human skills", which might well be the least actionable proposal ever suggested.
One of the relatively few other papers that reviews the market of 'A.I' scribes breezes past potential downsides, plainly concluding that burnout is a problem and AI scribes are the best solution. It is noted at the end that the author is the founder of a company that specialises in AI scribe services, but that this has, obviously, "not influenced the content of the manuscript".
The same pattern of uncritical reporting, poor examination of citations, and overly optimistic conclusions that handwave established issues continues in the rest of the literature on digital scribes, with studies finding unsolvable issues of significance and then counterbalancing these issues by churning out meaningless gunch like "it is through a combination of technological advancements, regulatory foresight, interdisciplinary collaboration, and educational efforts that the health care system can use AI effectively while addressing its potential drawbacks".
“In one example, the physician mentioned scheduling a prostate examination for the patient and the AI scribe summarized that a prostate examination had been performed. In another, the physician mentioned issues with the patient’s hands, feet, and mouth and the AI summary recalled the patient being diagnosed with hand, foot, and mouth disease. There were also a few instances where the summary was missing some details, such as missing chest pain and anxiety assessments."
—A study that still, bafflingly, concludes digital scribes should be integrated directly into health records: Ambient Artificial Intelligence Scribes to Alleviate the Burden of Clinical Documentation.
It really does just go on, and on, and on. I have a document full of links to studies that seem dubious: at least three times as many as we've covered. I'm so tired. I feel like I've been shifted into a world where fundamental assumptions about risk are totally different to the ones that everyone has broadly agreed upon for quite a long time.
This is Not The Future
This has been the sort of article to write where, every step of the way, I've asked myself what the point I'm trying to get across is.
Ultimately, I think, it's this: There is a veneer of 'intellectual value' given to scientific literature, doubly so for anything on complex topics. All I've been trying to do is scrape it off and show, in some way, that the unvarnished cabinetry of 'A.I science literature' is held together with rotten, mouldering particleboard. You do not need to sit in vaguely confused awe; it's shit and not fit for purpose.
There are very effective uses for complex machine learning. This is not a new form of technology. We've been using this stuff to do shit like identify whales from low-resolution pictures of their tail patterns for yonkers. I love classifiers in research. I love random and procedural generation. I love the sheer scale of hubris inherent to the idea that 'if we cram enough statistics into this hard drive we can teach it to write mid-tier erotic poetry on command'. I am not above tormenting my friends with badly generated images of Sonic the Hedgehog on the toilet.
Nonetheless, I do not think any of that shit is ready to be in healthcare. It may never, ever be, and I'm sick of, functionally, seeing BASE jumping proposed as an alternative to one of the safest examples of heavy machinery we have, as a society, ever invented.
Databases are not going to, ever, spontaneously tell you to kill yourself. This is the kind of reliability I want in medical software, and this is the kind of reliability we all want. If anyone claims otherwise I'm going to break into their home and reprogram every single bit of tech they own to diagnose them with horrible diseases at random intervals. Even the dishwasher.
These problems with machine learning are not going to vanish. The industry is going to break. Despite the hype, it is important that more people understand how badly the industry is stalling. Generative machine learning, and machine learning models in general, are turning out to be not-all-that-viable for most things. There will be a last, desperate dash to milk the last few fever dollars from whatever rubes they can find.
The entire industry is hitting several very firm walls at once (for instance, training and running models at an industry scale simply produces so much heat that it is, on a physical level, very hard to keep the hardware cool enough to function). In fact, the industry has been struggling to bypass a slew of issues for all of 2024, all while cheerfully insisting that progress has never been faster.
When it comes to summing up the industry, all the assembled studies I could dredge up can't paint a clearer picture than Edward Zitron, who I have quoted before and will quote again, and there's not a damn thing you meddling kids can do about it.
"I have been warning you for the best part of a year that generative AI has no killer apps and had no way of justifying its valuations, that generative AI had already peaked, and I have pleaded with people to consider an eventuality where the jump from GPT-4 to GPT-5 was not significant, in part due to a lack of training data.
I shared concerns in July that the transformer-based-architecture underpinning generative AI was a dead end, and that there were few ways we'd progress past the products we'd already seen.
Throughout these pieces I have repeatedly made the point that — separate to any lack of a core value proposition, training data drought, or unsustainable economics — generative AI is a dead end due to the limitations of probabilistic models that hallucinate, where they authoritatively state things that aren't true."
—Edward Zitron, in 'Godot Isn't Making It' on his website, Where's Your Ed At?
There has, as Ed points out, been a persistent trend of the media and business at large taking as 'gospel' the idea that machine learning and neural networks will just 'scale'; the more resources given, the better they will become. They will not just get more efficient, but also unlock new capabilities!
This has not played out, and the industry is bleeding money as it operates at a loss and pursues outlandishly ambitious increases in hardware power for very little benefit, all while being hyped to the world by flagrant liars.
"Anthropic CEO Dario Amodei said that "AI-accelerated neuroscience is likely to vastly improve treatments for, or even cure, most mental illness," [which is] the kind of hyperbole that should have you tarred and feathered in public."
—Edward Zitron, again in 'Godot Isn't Making It' on his website, Where's Your Ed At?
If you want a comprehensive breakdown of the technical and logistical issues at play, an overview of the scale of the money and resources being thrown at unsolvable problems, or the sheer hubris of the people in charge of these decisions, read Ed Zitron's work, starting with the linked article, Godot Isn't Making It.
As for me, I think I'm done. If you see any use of the term "AI" in any real-world business from now on, you should be filled with a deep sense of well-cited dread. You should think "that sounds like adding BASE jumping to my daily commute".
Still, though, I am sitting here after my eighth or ninth round of revisions, thinking "am I being detailed enough? Have I been specific enough about the problems in these studies? Have I shown enough examples?"
The answer, of course, is that at some point I have to publish this bloody thing before I accidentally put too many words (training data) in this text file (language model) and it gains sentience and eats me for nutrients (this is how machine learning works).